The Three-E Triangle – Training Tradeoffs
Reza Jalaeian discusses common challenges instructional designers face when creating effective, efficient, and engaging instruction and how the ShadowBox method can aid in attaining all three.
Project managers in industry may be familiar with the Project Triangle concept, which represents the triple constraints of Time, Cost, and Quality. The concept is that a client must choose two constraints to prioritize – at the cost of the third constraint:
- If the client needs the project to be fast & cheap, it won’t be good QUALITY
- If the client needs the project to be cheap & good quality, it won’t be FAST
- If the client needs the project to be fast and good quality, it won’t be CHEAP
Although this concept has been challenged in various ways, the idea remains and is commonly represented by a triangular diagram.
Inspired by the project triangle, instructional designers advocated for a similar approach to training and instruction by representing three common priorities. The First Principles of Instruction (FPI) (Merrill, 2008) asserts that good instruction/training should be effective, efficient, and engaging (e3) and be based on tasks that fit together to solve real-world problems.
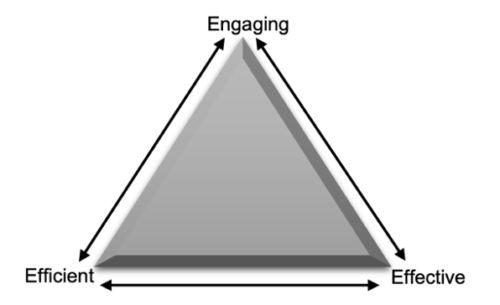
Together, these priorities provide clear goals for creating optimal training programs. However, in many performance domains, it remains a constant challenge for researchers and practitioners to create e3 training programs that can somehow accelerate the development of performers to higher levels of expertise.
Malcolm Gladwell (2008), in his popular work Outliers, discusses the notion of 10,000 hours or 10 years of deliberate practice to achieve expertise in a specific domain. Deliberate practice, as defined by Ericsson (2003), involves repetition and immediate feedback, which is typical for learners to achieve expertise in complex perceptual-cognitive and motor skills such as music and sports. But it is also relevant in other domains, especially professions such as the military, law enforcement, and healthcare where the role and form of deliberate practice is less clear (Fadde & Jalaeian, 2019). By extending the deliberate practice principles into these professions and applying them to the aspects of expertise, such as recognition and decision-making (Klein, 1997, 1999, 2009), which are typically assumed to come only with massed experience, we can accelerate the development of expertise. Klein’s Recognition Primed Decision Making (RPD) model describes how high stakes decision making, as an important aspect of expertise, is usually carried out in real-world settings. The model shows that experts use their experience to make decisions by quickly assessing the situation to generate a plausible course of action and then running mental simulation to evaluate that course of action (Klein, 1993). Such skills can be trained by setting up practices that allow trainees to see the world through the eyes of experts (Klein, Hintze, & Saab, 2013).
However, it appears increasingly difficult to accumulate these hours and years of deliberate practice to improve decision making skills. This could be one reason, as Ericsson (2014) suggests, that we do not have as many experts in different domains as we should. Simply put, fewer people have the time and/or motivation to practice while in the line of duty. On the other hand, expertise is perishable, and due to the increasingly complex world, the bar for mastery is higher. So, it is hard to achieve a level of mastery in the first place and hard to maintain it because mastery is a moving target. In addition, while some domains, such as sports and music, have a strong practice culture, other domains, such as law enforcement, military, aviation, and healthcare, may not necessarily have a culture of practice, which is yet another challenge in creating effective, efficient, and engaging training regimens that provide accessible practice for learners to move them up the ladder of expertise.
Although high-fidelity simulations such as virtual reality (VR) and augmented reality (AR) are areas of strong interest that could bring high levels of realism into training centers, which is great, they could be costly, time consuming, and less accessible for trainees. Moreover, and more importantly, it remains an open question whether such sophisticated training technologies, at least as actually employed, can improve the performance by a margin that is considerably higher in comparison to their less technologically enhanced counterparts. The assumption is that a higher level of fidelity equals better training, but I believe that is an assumption worth questioning through empirical and/or experimental studies.
So, what do we need to do to address at least some of these challenges? As mentioned above, one of the major aspects of expertise and higher levels of performance in almost all domains is the ability to make good decisions quickly and under stress. One method that allows trainees to practice their decision-making skills and learn from an expert without an actual expert being present and in a highly accessible environment is the ShadowBox training method first invented by Neil Hintze and further developed by Gary Klein and associates. This method has been employed effectively in domains such as social services, law enforcement, healthcare, and the military, with results revealing revealed significant improvement in student performance from pre- to post-test (Klein & Borders, 2016). Using a simple web-based technology that strives for conceptual over physical fidelity in training scenarios, the ShadowBox method is highly efficient by providing easy and affordable access to learners to polish their decision-making skills while engaging with realistic scenarios.
In today’s age of rapidly changing technology and knowledge, stakeholders and leadership find it increasingly challenging to keep their employees up to required levels of competence. Although, high-fidelity simulators like VR and AR are alluring technologies that provide rich situational context for learners, we must have more accessible and yet more affordable training options that are proven to be working. The goal is to create a consistent culture of practice for our learners to accumulate the amount of deliberate practice they need and as a result to accelerate their rise to expertise. Therefore, we need more task-based and more problem-centered types of training that put greater emphasis on quality of instruction rather than merely method of delivery. This is an important element of training for decision-making skills that has not received nearly enough attention.
For more information on how ShadowBox can provide more efficient, effective, and engaging training:
References
Ericsson, K. A. (2003). Development of elite performance and deliberate practice: An update from the perspective of the expert performance approach. Expert performance in sports: Advances in research on sport expertise, 49-84.
Ericsson, A., Prietula, M., & Cokely, E. (2014). The Making of an Expert. Harvard Business School Publishing.
Fadde, P., & Jalaeian, M. (2019). Learning with Zeal: From Deliberate Practice to Deliberate Performance. In J. M. S. Paul Ward, Julie Gore, and Emilie M. Roth (Ed.), The Oxford Handbook of Expertise: Oxford University Press.
Gladwell, M. (2008). Outliers: The story of success. Little, Brown.
Klein, G. A. (1993). A recognition-primed decision (RPD) model of rapid decision making. Decision making in action: Models and methods, 5(4), 138-147.
Klein, G. (1997). Developing expertise in decision making. Thinking & Reasoning, 3(4), 337-352.
Klein, G. (1999). Sources of power: How people make decisions. Cambridge, MA: MIT Press.
Klein, G. (2009). Streetlights and shadows: Searching for the keys to adaptive decision making.
Klein, G., & Borders, J. (2016) The ShadowBox approach to cognitive skills training: An empirical evaluation. Journal of Cognitive Engineering and Decision Making, 10 (3), pp.268–280.
Klein, G., Hintze, N., & Saab, D. (2013). Thinking inside the box: The ShadowBox method for cognitive skill development. In Proceedings of the 11th International Conference on Naturalistic Decision Making (pp. 121-124).
Merrill, M. D. (2008). Converting e3-learning to e3-learning: An alternative instructional design method. In S. Carliner & P. Shank (Eds.), The e-Learning Handbook: Past Promises, Present Challenges (pp. 359-400). San Francisco: Pfeiffer.